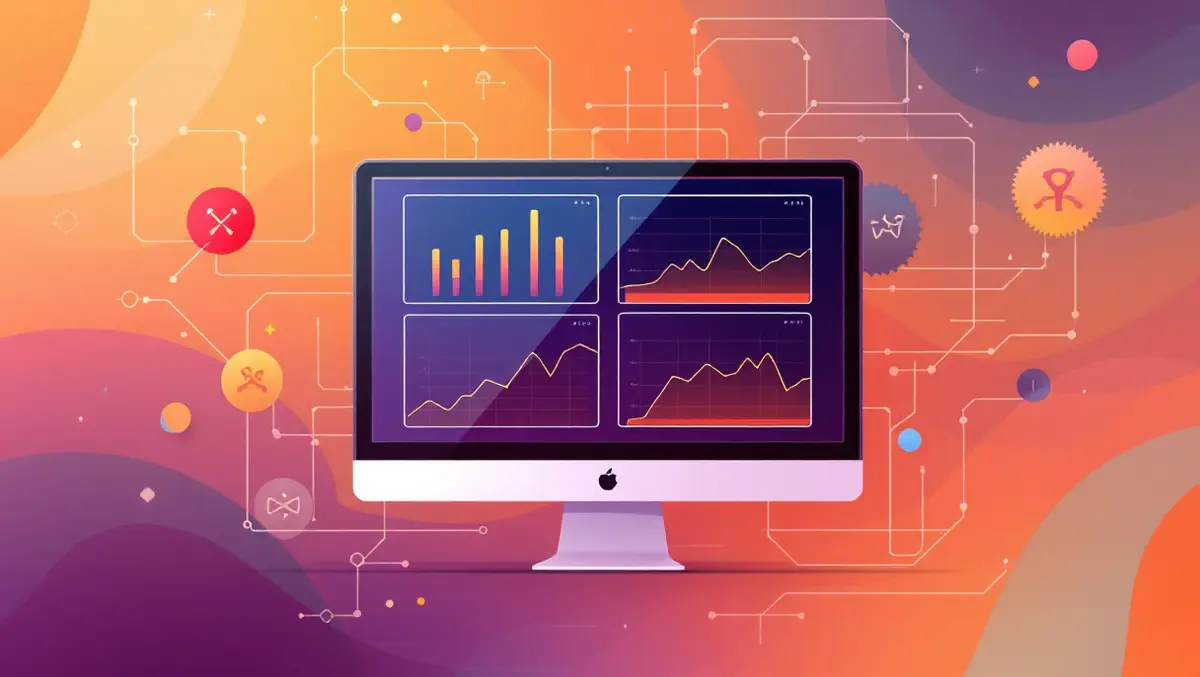
Datadog unveils open-source AI models for better observability
Datadog has announced the release of two artificial intelligence foundation models through its AI research lab, aimed at advancing anomaly detection and analysis in machine learning and observability.
The two projects unveiled are Toto, an open-weights, zero-shot time series foundation model, and BOOM, described as the largest public benchmark of observability metrics to date. Both are intended to support the broader research community through open-source access and published findings.
Datadog AI Research is focused on bridging the gap between academic developments and practical implementation within the domains of cloud observability and security. The company states that it will regularly contribute to the research ecosystem by making research outputs and model artefacts accessible to researchers, developers and engineers worldwide.
Toto is the first open-source foundation model created specifically for observability purposes. It is designed to function as a time series foundation model (TSFM), learning from large datasets to be adaptable across numerous forecasting and anomaly detection tasks—similar in concept to large language models, but applied to telemetry data. Its training relied exclusively on Datadog's internal telemetry metrics, which the company says enables it to outperform other TSFMs on a range of tasks.
According to Datadog, Toto's "zero-shot" design allows it to deliver immediate forecasts and anomaly detection without requiring tuning for each new data series. This quality, the company notes, is particularly valuable when monitoring systems generate billions of individual time series that change frequently. While existing TSFMs reportedly face challenges handling the complexities of telemetry data, the introduction of Toto is claimed to offer improved performance both for observability and general forecasting objectives.
BOOM, the second release from the lab, provides a new benchmark targeted specifically at observability metrics, which often display characteristics such as high sparsity, sudden spikes and cold-start scenarios that differ from standard time series data. The benchmark comprises 350 million observations across 2,807 real-world multivariate series, reflecting the scale and variety often encountered in production telemetry environments. Datadog submits that BOOM will serve as a resource for researchers attempting to advance forecasting models suitable for observability use cases.
"Today marks the launch of our first open-source foundation model and we expect to continuously release AI projects through Datadog AI Research," said Ameet Talwalkar, Chief Scientist at Datadog. "The lab offers an exciting opportunity to develop research ideas and build prototypes that will contribute to the community. We will also collaborate with applied AI teams to build tools that will solve customer problems and transform how engineers work."
Datadog has stated that the new lab will work closely with its product and engineering teams to ensure that the advances stemming from projects such as Toto and BOOM are translated into practical benefits for customers using its monitoring and security platform.
Both Toto and BOOM are immediately available for download under a permissive open-source licence. Datadog is encouraging researchers and members of the open-source software community to use and improve upon these tools to further observability forecasting research.
Recently, Datadog expanded its AI monitoring capacity with the acquisition of Metaplane, an AI-powered observability start-up. This acquisition is intended to bolster the company's capabilities in providing reliable and robust AI systems for business customers.